News Story
Director Editorial: Machine Learning and HVAC
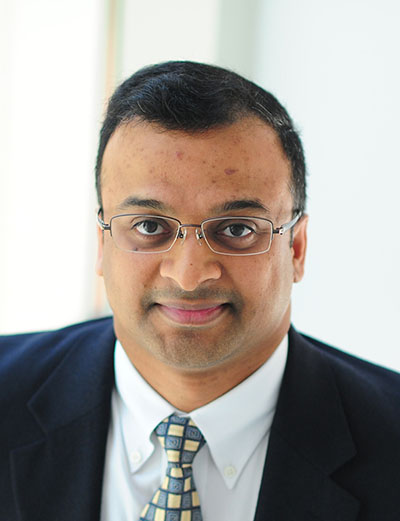
Dr. Vikrant Aute
Machine Learning (ML) encompasses several techniques, some of which, e.g., regression, were developed more than two centuries ago (Stigler, 1981). Other methods, such as neural networks, came about in the early 19th century. But it was not until the 2010s that the term became an everyday buzzword. The critical enablers for this were the widespread availability of algorithms and computing hardware. The low-cost hardware and free algorithms have enabled the generation, storage, and processing of massive amounts of data. A notable example highlighting the ease of use of ML is that of a farmer (Sato, 2016) using ML implemented on commodity hardware to sort cucumbers for specialized packing and sale. ML is generally used in conjunction with Big Data or Data Analytics. And this is where businesses and organizations can leverage ML and analytics to automate business decisions, generate new business opportunities, and provide customer value.
While many companies are already using ML for the analysis of deterministic data, there is little published evidence of breakthrough ML applications in the HVAC&R industry. The research at CEEE has been leveraging ML techniques since 2005.
As this proliferation and democratization of ML continue, what does it mean for the HVAC&R industry? The following thoughts come to mind.
Data Collection: Good data is critical for the success of any ML effort. Any HVAC unit in the field that is not instrumented and gathering data is a lost opportunity. The analysis objectives and framework need not be in place for this.
Rapid Design and Design Assistants: One area where ML will revolutionize HVAC&R systems is the way in which they are designed. ML will allow rapid exploration of design space at the component and system level leading to significantly advanced designs that were never intuitive before. It could also include system synthesis, i.e., ML would invent the process or the cycles. We are beginning to see the foundational work for this in the literature now. Design exploration will be augmented by virtual design assistants enabling significant productivity boosts for engineers. Even with these virtual assistants, the need for subject matter experts is not going away. CEEE is working towards developing such a design assistant for heat pump components and systems.
Next Generation Controls: ML is expected to mark the end of traditional controls as we know them. Traditional control schemes are often based on fixed sensors & actuators and a deterministic interaction between a system and its ambient. But ML-based controls can discern subtle variations in system behavior through sensor readings and can adapt, leading to improved operations and energy savings (Knight, 2018).
Democratization of ML and AutoML: Most sophisticated ML algorithm implementations are freely available and/or are being developed by non-HVAC organizations. More recently, platforms like AutoML allow one to conduct analytics automatically on a given data, further reducing the barrier to entry for leveraging ML.
Crowdsourcing of ML: Platforms like Kaggle allow organizations to jumpstart their ML efforts through crowdsourcing. Organizations can share anonymized data sets, specify targets, and seek ML solutions, many of which could potentially be free (code and scripts, including visualizations).
It wouldn’t be farfetched to envision a future wherein we could call upon our favorite digital assistant and ask: “Give me the lowest cost heat exchanger for…”.
Current research at CEEE is laying the foundations for such a virtual design assistant.
References:
- Proceedings of the Silicon Valley AI Summit, 2020.
- Knight, W. (2018, August 17). Google just gave control over data center cooling to an AI. https://www.technologyreview.com/2018/08/17/140987/google-just-gave-control-over-data-center-cooling-to-an-ai/
- Sato, K. (2016, August 31). How a Japanese cucumber farmer is using deep learning and TensorFlow. Retrieved from https://cloud.google.com/blog/products/gcp/how-a-japanese-cucumber-farmer-is-using-deep-learning-and-tensorflow
- Stigler, 1981, Gauss and the Invention of Least Squares, The Annals of Statistics, Vol. 9. No 3.
Published December 9, 2020