News Story
CEEE Study Explores How AI Can Reduce HVAC Energy Consumption
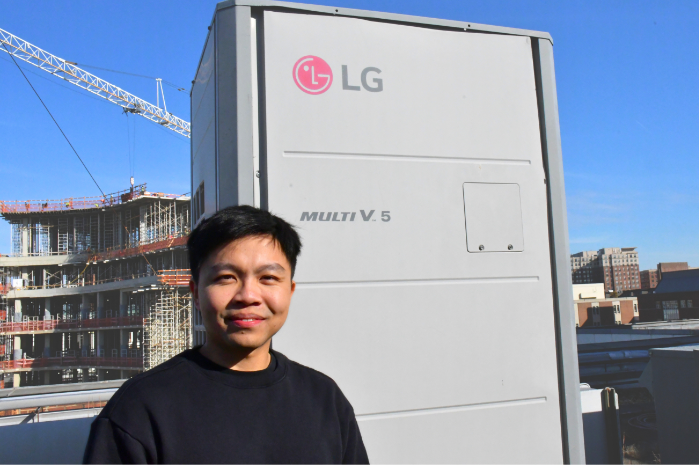
Graduate research assistant Po-Ching Hsu stands on the rooftop of Glenn L. Martin Hall with the outdoor HVAC unit used for a field test that compared two different types of AI models in reducing energy consumption in variable refrigerant flow systems.
A sophisticated type of artificial intelligence (AI) known as deep learning could play an important role in reducing energy usage in the next generation of heating, ventilation and air conditioning (HVAC) systems, according to research findings recently published by the University of Maryland Center for Environmental Energy Engineering (CEEE).
The researchers explored AI’s impact on predicting power consumption in variable refrigerant flow (VRF) technology – complex HVAC systems that have an outdoor unit and multiple indoor units – and presented their findings in the January 2025 issue of the “International Journal of Refrigeration,” now available online.
HVAC accounts for around 50% of a building’s total electricity consumption, the paper reports, so finding ways to slash HVAC energy consumption could significantly decrease a building’s overall energy usage. Energy consumption predictions are used to help optimize a VRF system’s efficiency and thermal comfort.
The researchers compared two types of AI models – a traditional machine learning model known as Artificial Neural Network (ANN) and a more recently developed deep learning model called Long-Short-Term Memory (LSTM). Both models use data to recognize patterns and produce insights and predictions – with LSTM requiring a larger data set. In this case, the models looked at data gathered from a VRF system installed at the university’s Glenn L. Martin Hall, taking into account variables like indoor and outdoor temperatures and humidity levels. Data was taken for a period of a year, so as to cover all four seasons.
As expected, the team found that the more data-intensive LSTM model had better accuracy in predicting power consumption. The big surprise, though, was that the LSTM model appears to require less computing power and memory than the ANN model. “That was counterintuitive,” said lead author mechanical engineering graduate student Po-Ching Hsu, who had hypothesized that the more sophisticated LSTM model would require more computing power. “But, what happens is that the ANN tries to improve its accuracy during the optimization process,” he explains. “So it keeps increasing the parameters inside the structures in order to have a more complex model to predict the power consumption. But even with that, it still cannot achieve the same performance as LSTM.”
The optimized ANN model had over 13,500 trainable parameters, while the LSTM model had 1,809 trainable parameters. “A higher number of trainable parameters in models is likely to result in increased memory usage on the computer and be computationally expensive,” the paper notes.
The paper’s other co-authors are former CEEE researcher Lei Gao Ph.D. ’22, now on the R&D staff at Oak Ridge National Laboratory, and CEEE Co-Director Yunho Hwang, a mechanical engineering research professor.
Deep learning could be a powerful tool in improving the energy efficiency of VRF systems, says Hsu, but the challenge is decreasing the time required to gather sufficient data. “After the AC system is installed, it would need to collect data for one year to become optimized,” says Hsu. “We are trying to figure out if we can train this model with less data,” he says. “Is there a way we can do this with data from a few days or a few weeks and still make very good predictions?”
Download the paper: “Comparative study of LSTM and ANN models for power consumption prediction of variable refrigerant flow (VRF) systems in buildings.” The full paper is available for free download until December 19, 2024.
Published November 5, 2024